Significantly Statistical Methods
a Free Online Statistics Course with JMP Software
Interested in learning about statistical inference, data analysis, and the philosophy of science? If so, this free online course is for you!
No previous statistical experience is necessary, and a 30-day trial for JMP to accompany this course is available at jmp.com/try. Start learning today!
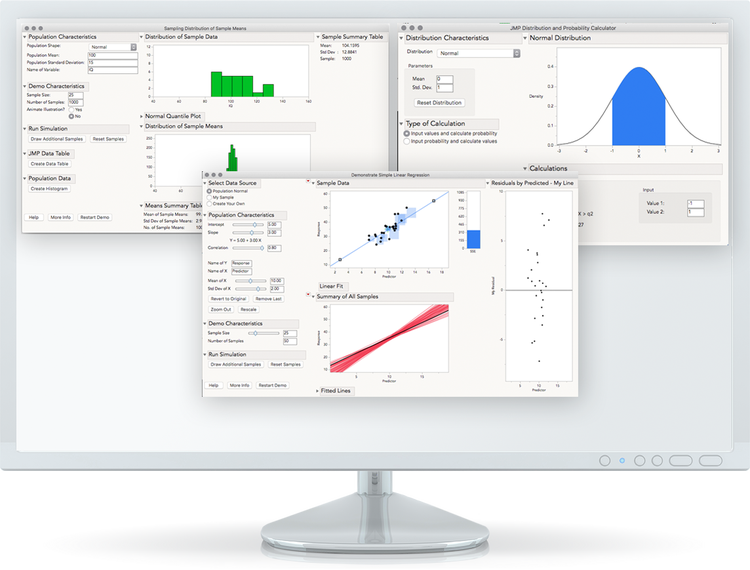
Unit 1 - Theory of Statistics
Unit 2 - Linear Models of Means
Statistics is more than just math, and it's more than just a collection of methods to analyze data; statistics provides a way to think about the world in a principled fashion, to interpret the outcomes of events unfolding before us, to see the structure of things amidst the prevailing noise and randomness of our reality. If you work with data right now, or want to start a career where you will, there is nothing more valuable than a solid foundation in statistical methods.
Statistics, by its very nature, is cumulative; a thorough understanding of basic topics allows for more complicated concepts to be developed. For this reason, I strongly recommend watching the videos in this course sequentially. If you have previous experience with statistics you may find value in skipping to a particular topic of interest; but, you will gain the most value from the content here by following the course as it is presented.
In Unit 1 I introduce the fundamental concepts of sampling distributions, statistical error, and statistical inference. These concepts form the foundation of the remainder of the course. In Unit 2 we will discuss mathematical models for statistical inference and how these models allow us to decide whether or not we have statistical evidence for differences between groups. Unit 3 will extend these mathematical models to situations where individuals differ not in terms of a group, but in terms of the quantitative amount of some "predictor" we have measured or assigned.
Take your time with this course and apply as often as possible the concepts we cover. Like training for a marathon, learning statistics is not something you should rush. Many of the concepts in this course require time to fully understand, so expect to spend several weeks to several months to complete this course.
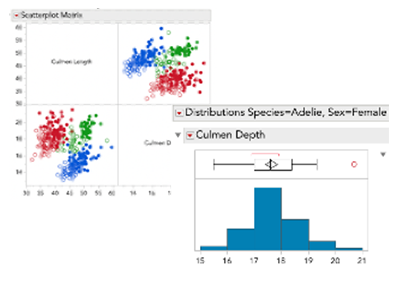
Julian Parris is the Director of Research and Development at JMP Statistical Discovery.
In this role, Parris leads the department responsible for data table, scripting, display, graphics, and many other foundational parts of JMP. Prior to heading R&D, she led JMP’s User Acquisition team, created SKP (the Statistics Knowledge Portal) and managed JMP’s Strategic Initiatives and Analytics group, just to name a few of her many contributions to the company.
Before joining JMP, Parris was a professor in the Department of Psychology at the University of California, San Diego (UCSD) where she used JMP to teach intermediate and advanced statistics and research methods to students in the social sciences.
She holds both a master’s degree and Ph.D. in experimental psychology from UCSD, where she studied various aspects of human behavior as they relate to interactions with computers, evidence, and inference. She holds a bachelor’s degree in psychology from UCLA.
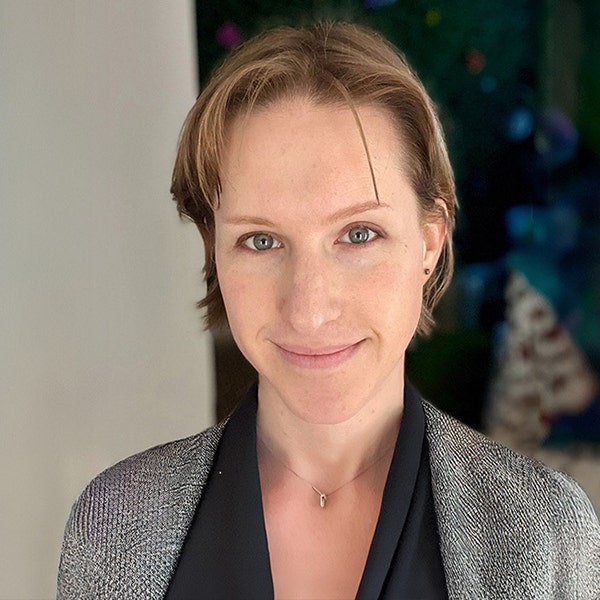
Unit 1 - Theory of Statistics
Module 1:1 - Introduction to Science and Data
Module overview
Concept Keywords
Introduction, Methods of Knowing, The Criterion of Falsifiability, The Scientific Method, Correlational Studies, Manipulation in Experimental Design, Control in Experimental Design, Correlational Methods of Research, Experimental Methods of Research, Basic Terminology, Sampling Error and Explanations in Science, Measurement Theory, Constructs and Operational Definitions, Scales of Measurement, Qualities of Measurement, The Anatomy and Interface of JMP, Saving and Sharing Work in JMP
Sections
- Introduction to Significantly Statistical Methods in Science - 5:07
- Methods of Knowing - 7:38
- The Criterion of Falsifiability - 6:36
- The Scientific Method - 3:47
- Correlational Studies - 4:22
- Manipulation in Experimental Design - 9:25
- Control in Experimental Design - 4:20
- Correlational Methods of Research - 2:22
- Experimental Methods of Research - 8:15
- Basic Terminology - 9:45
- Sampling Error and Explanations in Science - 8:15
- Measurement Theory - 2:25
- Constructs and Operational Definitions - 1:26
- Scales of Measurement - 12:51
- Qualities of Measurement - 3:13
- The Anatomy and Interface of JMP - 13:29
- Saving and Sharing Work in JMP - 16:46
Module 1:2 - Visual Displays of Data
Module overview
Concept Keywords
Frequency Distributions, The Shape of Data in JMP, Graph Builder Basics in JMP
Sections
Module 1:3 - Quantifying Distributions
Module overview
Concept Keywords
Central Tendency, Visualizing the Mean, Introduction to Variability, Deciding on an estimator for the population variance, Estimating the Population Variance, Simulating Variance Estimates
Sections
Module 1:4 - Quantifying Locations
Module overview
Concept Keywords
Describing Locations of Scores in Distributions, Intro, Seeing the locations of scores in a distribution with JMP, Developing the Z-score, Using JMP to Standardize Scores, Characteristics and Uses of Z-scores
Sections
Module 1:5 - Probability and The Normal Distribution
Module overview
Concept Keywords
Basics of Probability, Intro, Basic Definitions of Probability, Exploring Probability in JMP with a deck of cards, Exploring Probability in JMP with rolling dice, Exploring the probability of randomly selecting individuals in JMP, 1, Exploring the probability of randomly selecting individuals in JMP, 2, The Normal Distribution
- Basics of Probability, Intro - 0:59
- Basic Definitions of Probability - 8:08
- Exploring Probability in JMP with a deck of cards - 4:51
- Exploring Probability in JMP with rolling dice - 4:59
- Exploring the probability of randomly selecting individuals in JMP, 1 - 4:36
- Exploring the probability of randomly selecting individuals in JMP, 2 - 6:38
- The Normal Distribution - 5:52
Module 1:6 - Sampling Distributions
Module overview
Concept Keywords
Sampling Distributions, Intro, Binomial Sampling Demo 1, Binomial Sampling Demo 2, Binomial Sampling Demo 3, Distribution of Sample Means, Intro 2, Sampling Distribution Definitions, and Making a Real Sampling Distribution, Sampling Distribution Simulation with a Large Population, n=2, Sampling Distribution Simulation with a Large Population, larger samples, Characteristics of Sampling Distributions, and the Standard Error, The Supreme Law of Unreason, The Central Limit Theorem
Sections
- Sampling Distributions, Intro - 2:16
- Binomial Sampling Demo 1 - 8:32
- Binomial Sampling Demo 2 - 6:14
- Binomial Sampling Demo 3 - 7:28
- Distribution of Sample Means, Intro 2 - 9:40
- Sampling Distribution Definitions, and Making a Real Sampling Distribution - 9:16
- Sampling Distribution Simulation with a Large Population, n=2 - 10:15
- Sampling Distribution Simulation with a Large Population, larger samples - 6:52
- Characteristics of Sampling Distributions, and the Standard Error - 11:43
- The Supreme Law of Unreason, The Central Limit Theorem - 3:52
Module 1:7 - Statistical Inference I
Module overview
Concept Keywords
Sampling Distributions and Statistical Inference, The Logic of Hypothesis Testing, Prediction and Standard of Evidence, Test Statistic, Evaluation, Z-test In JMP
Sections
- Sampling Distributions and Statistical Inference - 13:22
- The Logic of Hypothesis Testing - Hypotheses I - 6:35
- The Logic of Hypothesis Testing - Hypotheses II - 3:55
- The Logic of Hypothesis Testing - Hypotheses III - 4:39
- The Logic of Hypothesis Testing - Prediction and Standard of Evidence I - 4:20
- The Logic of Hypothesis Testing - Prediction and Standard of Evidence II - 4:54
- The Logic of Hypothesis Testing - Test Statistic - 2:13
- The Logic of Hypothesis Testing - Evaluation I - 2:52
- The Logic of Hypothesis Testing - Evaluation II - 2:28
- The Logic of Hypothesis Testing - Z-test In JMP - 2:25
Module 1:8 - Statistical Inference II
Module overview
Concept Keywords
Hypothesis Testing Decisions, Probability of Errors, The Strength of Decisions, Never Accept The Null Hypothesis, Visualizing Statistical Power, Statistical Power, Effect size, Variability, Sample Size, Alpha Level, Directional Hypotheses
Sections
- Hypothesis Testing Decisions I - 7:22
- Hypothesis Testing Decisions II - Probability of Errors - 4:22
- Hypothesis Testing Decisions III - The Strength of Decisions - 5:56
- Never Accept The Null Hypothesis - 4:51
- Visualizing Statistical Power I - 6:19
- Visualizing Statistical Power II - 6:09
- Factors Affecting Power - Effect size, Variability, Sample Size - 8:10
- Factors Affecting Power - Alpha Level - 3:14
- Factors Affecting Power - Directional Hypotheses - 11:52
- Factors affecting Power wrap-up - 3:27
Module 1:9 - Basic Hypothesis Testing with t
Module overview
Concept Keywords
Introducing the t-statistic, One-Sample t-test, Logic and JMP, Developing the dependent measures t-test, The dependent measures t-test in JMP I, The dependent measures t-test in JMP II, Developing the independent measures t-test, The independent measures t-test in JMP I, The independent measures t-test in JMP II, Advantages and Concerns with Repeated Measures Designs, Deciding on a hypothesis test tree
Sections
- Introducing the t-statistic - 4:31
- One-Sample t-test, Logic and JMP - 7:05
- Developing the dependent measures t-test - 8:26
- The dependent measures t-test in JMP I - 3:46
- The dependent measures t-test in JMP II - 3:24
- Developing the independent measures t-test - 8:25
- The independent measures t-test in JMP I - 2:35
- The independent measures t-test in JMP II - 2:23
- Advantages and Concerns with Repeated Measures Designs - 8:12
- Deciding on a hypothesis test tree - 2:37
Unit 2 - Linear Models of Means
Module 2:1 - Linear Models
Module overview
Concept Keywords
Functional Relationships, Statistical Relationships, Components of the One Factor Linear Model, The Distribution of Error in the One Factor Linear Model, The One Factor Linear Model - Sample Form, Estimating the Mean Squared Error, Inference about treatment effect from the one factor linear model
Sections
- Introduction to Mathematical Models - Functional Relationships - 8:05
- Introduction to Mathematical Models - Functional Relationships II - 3:20
- Introduction to Mathematical Models - Statistical Relationships - 9:24
- Components of the One Factor Linear Model I - 7:23
- Components of the One Factor Linear Model II - 3:21
- The Distribution of Error in the One Factor Linear Model - 3:50
- The One Factor Linear Model - Sample Form - 7:54
- Estimating the Mean Squared Error - 8:00
- Inference about treatment effect from the one factor linear model - 2:28
Module 2:2 - ANOVA and the General Linear Test
Module overview
Concept Keywords
The Fisher-Snedecor Distribution and the analysis of variance, The Analysis of Variance Test Statistic, Partitioning the Sums of Squares in ANOVA, The Sums of Squares Treatment in ANOVA, Degrees of Freedom in One Factor ANOVA, The General Linear Test
Sections
- The Fisher-Snedecor Distribution I - 6:00
- The Fisher-Snedecor Distribution II - 5:25
- The Fisher-Snedecor Distribution and the analysis of variance - 2:40
- The Analysis of Variance Test Statistic - 5:17
- Partitioning the Sums of Squares in ANOVA - 6:59
- The Sums of Squares Treatment in ANOVA - 2:15
- Degrees of Freedom in One Factor ANOVA - 6:06
- The General Linear Test I - 3:46
- The General Linear Test II - 7:29
- The General Linear Test III - 4:07
Module 2:3 - ANOVA and pairwise comparisons in JMP
Module overview
Concept Keywords
One-Factor ANOVA in JMP with Fit Y by X, One-Factor ANOVA in JMP with Fit Model, Pairwise Comparisons in JMP with Fit Y by X, Pairwise Comparisons in JMP with Fit Model, The Problem of Multiplicity and Alpha Escalation, Controlling Alpha for Planned Comparisons, Controlling Alpha for Unplanned Comparisons, Multiple Comparison Summary
Sections
- One-Factor ANOVA in JMP with Fit Y by X - 5:59
- One-Factor ANOVA in JMP with Fit Model - 9:00
- Pairwise Comparisons in JMP with Fit Y by X - 9:03
- Pairwise Comparisons in JMP with Fit Model - 3:15
- The Problem of Multiplicity and Alpha Escalation I - 3:19
- The Problem of Multiplicity and Alpha Escalation II - 2:45
- Controlling Alpha for Planned Comparisons - 6:27
- Controlling Alpha for Unplanned Comparisons - 4:41
- Multiple Comparison Summary - 1:37
Module 2:4 - Factorial ANOVA
Module overview
Concept Keywords
Introduction to Factorial Designs, The Three Possible Tests in a Two-Way Factorial Design, The Two-Factor Linear Model, Modeling the Cell Means in the Two Factor Linear Model, Estimating the Main Effects in the Two Factor Linear Model, Estimating the Interaction in the Two Factor Linear Model, The Structure of the Interaction Offsets in the Two Factor Linear Model, The Cell Means as a Function of the Row, Column, and Interaction Effects, Residuals and Test of Effects in the Two Factor Linear Model
Sections
- Introduction to Factorial Designs - 6:10
- The Three Possible Tests in a Two-Way Factorial Design - 6:31
- The Two-Factor Linear Model - 6:10
- Modeling the Cell Means in the Two Factor Linear Model - 6:11
- Estimating the Main Effects in the Two Factor Linear Model - 8:38
- Estimating the Interaction in the Two Factor Linear Model - 6:52
- The Structure of the Interaction Offsets in the Two Factor Linear Model - 4:48
- The Cell Means as a Function of the Row, Column, and Interaction Effects - 6:25
- Residuals and Test of Effects in the Two Factor Linear Model - 7:46
Module 2:5 - Factorial ANOVA in JMP
Module overview
Concept Keywords
Factorial Analysis Introduction, Defining the Model in Fit Model, Fit Model Output, Using Fit Model to Understand Effects, Prediction Profiler, Pairwise Comparisons in Fit Model, Pairwise Comparisons in Fit Model - Contrasts, Factorial ANOVA Larger than 2x2, Factorial ANOVA, Testing Slices in Factorial Designs
Sections
- Factorial Analysis Introduction - 1:23
- Factorial Analysis Introduction II - 2:50
- Factorial ANOVA - Defining the Model in Fit Model - 2:35
- Factorial ANOVA - Fit Model Output - 5:04
- Factorial ANOVA - Using Fit Model to Understand Effects - 4:06
- Factorial ANOVA - Prediction Profiler - 3:10
- Pairwise Comparisons in Fit Model - 5:07
- Pairwise Comparisons in Fit Model - Contrasts - 2:28
- Factorial ANOVA Larger than 2x2 - 1:27
- Factorial ANOVA - 10:05
- Testing Slices in Factorial Designs - 4:12
Module 2:6 - General Linear Model Assumptions
Module overview
Concept Keywords
Assumptions of the General Linear Model, Introduction, Homogeneity of Error for One Factor Models, Homogeneity of Error for Two Factor Models, Testing Homogeneity of Variance with Fit Y by X in JMP, Testing Homogeneity of Variance with Variability Gauge Chart
Sections
- Assumptions of the General Linear Model, Introduction - 3:28
- Homogeneity of Error for One Factor Models - 4:32
- Homogeneity of Error for Two Factor Models - 8:27
- Testing Homogeneity of Variance with Fit Y by X in JMP I - 2:50
- Testing Homogeneity of Variance with Fit Y by X in JMP II - 2:07
- Testing Homogeneity of Variance with Variability Gauge Chart - 4:43
Module 2:7 - Multifactor ANOVA
Module overview
Concept Keywords
Three Factor ANOVA, Data Quality Check before 3-Factor ANOVA, Using Fit Model in JMP to set up a Three-Factor ANOVA, Interpreting the Three-Factor ANOVA in JMP
Sections
Module 2:8 - One Factor Repeated Measures
Module overview
Concept Keywords
Introduction to Repeated Measures Designs and the One Factor Repeated Measures Model, Fixed and Random Factors, Visualizing the effect of modeling individual subject offsets, The Subject x Treatment Interaction Source as Error, Data Arrangements, Stacked and Split Data, Stacking and Splitting Data in JMP, Data Quality Check before Repeated Measures Analysis, Using Fit Model in JMP to set up a One factor Repeated Measures Analysis, Interpreting the Fit Model output in JMP for a One Factor Repeated Measures Analysis, Using the Repeated Measures Add-in for JMP, One Factor
Sections
- Introduction to Repeated Measures Designs and the One Factor Repeated Measures Model - 8:31
- Fixed and Random Factors - 5:57
- Visualizing the effect of modeling individual subject offsets - 5:17
- The Subject x Treatment Interaction Source as Error - 2:01
- Data Arrangements, Stacked and Split Data - 2:57
- Stacking and Splitting Data in JMP - 4:54
- Data Quality Check before Repeated Measures Analysis - 2:31
- Using Fit Model in JMP to set up a One factor Repeated Measures Analysis - 2:56
- Interpreting the Fit Model output in JMP for a One Factor Repeated Measures Analysis - 4:54
- Using the Repeated Measures Add-in for JMP, One Factor - 3:15
Module 2:9 - Factorial Repeated Measures
Module overview
Concept Keywords
Factorial Repeated Measures Model, Using the JMP Repeated Measures Add-In for Factorial Repeated Measures, Interpreting the Factorial Repeated Measures Output in JMP, Introduction to Repeated Measures with Between Subjects Factors, Using the JMP Repeated Measures Add-In for Mixed-Factor Repeated Measures, Interpreting the Mixed Factor Repeated Measures Output in JMP
Sections
- Factorial Repeated Measures Model - 4:18
- Using the JMP Repeated Measures Add-In for Factorial Repeated Measures - 4:43
- Interpreting the Factorial Repeated Measures Output in JMP - 2:23
- Introduction to Repeated Measures with Between Subjects Factors - 5:37
- Using the JMP Repeated Measures Add-In for Mixed-Factor Repeated Measures - 2:47
- Interpreting the Mixed Factor Repeated Measures Output in JMP - 6:29
Unit 3 - Linear Regression Models
Module 3:1 - Simple Linear Regression Models
Module overview
Concept Keywords
Introduction to Regression Models, The One Predictor Linear Regression Model, Least Squares, The Conditional Mean for Y given X
Sections
Module 3:2 - Simple Linear Regression Tests
Module overview
Concept Keywords
Error and Tests of Effect in Regression Models Module, T-Test for the SImple Regression Slope, T-Test for the Simple Regression Y-Intercept, Analysis of Variance Test of the Simple Regression Slope
Sections
Module 3:3 - Simple Linear Regression in JMP
Module overview
Concept Keywords
Simple Linear Regression in JMP with Fit Y by X, Simple Linear Regression in JMP with Fit Model